Have you ever wondered how climate scientists make climate projections? Global Climate Models are numerical models developed by climate scientists that run on powerful supercomputers. These models work by solving complex mathematical equations that help us understand how heat, moisture, and molecules move across space through time. Global climate models were traditionally used for research on climate systems, but they are also used to produce projections of future climate and thereby assists policymakers to make critical decisions in combating climate change. Scientists can also use climate models to study how climate change will affect specific regions or countries, to help people respond and prepare at a more local level.
Because global climate models represent the whole planet, they are much less reliable when applying them to only certain regions. Obviously, not every region on the planet is the same, nor will they be affected by climate change in the same way. To deal with this problem, scientists use a technique called statistical downscaling to translate global climate model projections to regional and local areas.
In addition, climate scientists previously make use of multiple global climate models at once, typically referred to as an ensemble. Climate scientists will often average the projections made by ensembles together into an ensemble mean. However, since multiple models may use similar algorithms, groups of models tend to cluster towards a particular result. When this happens, statisticians will say that the results have lost “independence.”
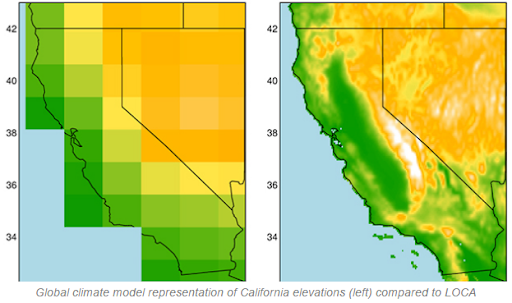
This is an example of what happens when you downscale a model. On the left, the model can’t really show with high precision what is going on in a specific part of California. The image on the right shows a downscaled projection — you can see much more detail! Source: USGS, Public Domain.
Independence is extremely important. But, what does it really mean for climate models to be “independent”? We can use an example from everyday life. If we ask a group of people how they think their work week will go, we expect their answers to be independent of each other, as everyone will have different things going on in their lives.
However, let’s say a significant number of them work at the same company. Their answers will not be independent, because they will be exposed to the same workplace events. Therefore the presence of a common background will over-represent that response in the average. In the same way, the presence of similar approaches to modeling tend to cluster towards a particular outcome.
When averaging multiple climate models together, whether global or regional, scientists also need to “weight” the climate models according to things like their historical accuracy and independence from other models. Weighting is just like how a professor would weigh a test more heavily than a homework assignment in a final grade using a percentage. This helps the researchers have more confidence in the accuracy of their climate projections.
The weights often used for averaging are developed using the global climate models themselves and not statistically downscaled climate projections. A team of researchers at University of Oklahoma and the NASA Jet Propulsion Laboratory wondered if this was a problem and performed simulations to determine if using weights from downscaled models instead of the usual global ones made any difference in the projections.
Their simulations used several widely-used weighting schemes along with past precipitation data from the south-central United States as a test case. They looked at how these different weighting schemes changed the projected rainfall in that region.
They performed calculations to choose climate models with the highest likelihood of matching historical observations when compared to other weighting schemes. Then each model was analyzed for how well it compared to real climate observations. The models were assigned weights based on these outcomes — the best matches were more heavily weighted.
A comparison of the weighted ensemble was made with the downscaled model and it was observed that the downscaled model had higher accuracy, but overall lower independence. l This observation demonstrates that statistical downscaling techniques have an influence on the accuracy of the climate predictions when multiple models are averaged together.
From the results, the researchers concluded that, on one hand, the accuracy of the models increases when downscaling is used. On the other hand, downscaling reduces independence, increasing the tendency of the models to cluster towards a particular outcome. These changes observed in accuracy and independence of the models upon downscaling challenge a long-held assumption that using weights from global models are the same as those from downscaled models, and thus don’t affect regional projections. It turns out, they do!
Do you like podcasts? There’s an episode of our podcast, Science Decoded, about this study! Check it out here. You can also find us on all your favorite streaming services, like Spotify, iTunes, iHeart Radio, PocketCasts, and Stitcher!